Making box plots was recently the topic of my livestream. I had been doing analytics on my YouTube channel, and it seemed that box plots and percentiles keep coming up as topics where learners are looking for answers. Therefore, I thought I’d put some practical information out there about making box plots – especially using R GUI.
I realized there are two main circumstances under which a data scientist would make a box plot (formally called box-and-whisker plot):
- She is in a hurry and wants to see the distribution of a quantitative variable on-the-fly while programming, so she runs a quick box plot, or
- She wants to present the box plot as final results in a report, so she makes a fancy one.
Basically, that’s what this blog post is about: Making box plots for these two different purposes.
Making Box Plots Scenario
Making box plots requires having a quantitative variable for which you are curious about the distribution. I find it hard to understand statistics without a real example, so I like to use public data from the American Hospital Directory (AHD.com) about hospitals, because people tend to understand hospitals in general.
For this blog post, I went to their list of hospitals in Massachusetts (where I live), and I copied the list of hospital names, and the number of staffed beds at each hospital. Just as a reminder, “staffed beds” means the number of inpatient beds the hospital has available for use for inpatient admissions. Hospitals are buildings with space, and they can use that space for many activities, including inpatient admissions – so the idea is that hospitals with more staffed beds are not only bigger, but they are doing more inpatient care delivery, which is the most expensive. If you want to know more about hospitals or healthcare finance, please take a look at these videos below.
I will show you the datasets below, but if you want them, you can download them from Github. I created an Excel *.xlsx file with two tabs – MAHosp and CityCompare – with the data on them. Then, I saved each dataset as a *.csv to read into R. Github wouldn’t let me upload the Excel file for some reason, so I just put the *.csvs in Github. I just find it easier to do it that way. I can groom the dataset in Excel – add formatting, freeze panes, and so on – and then just regenerate the *.csv when I want to put it in R. I admit, I had to do some work in Excel to get R to recognize my StaffedBeds variable as an integer. You will see in the livestream link one way to work through issues like this.
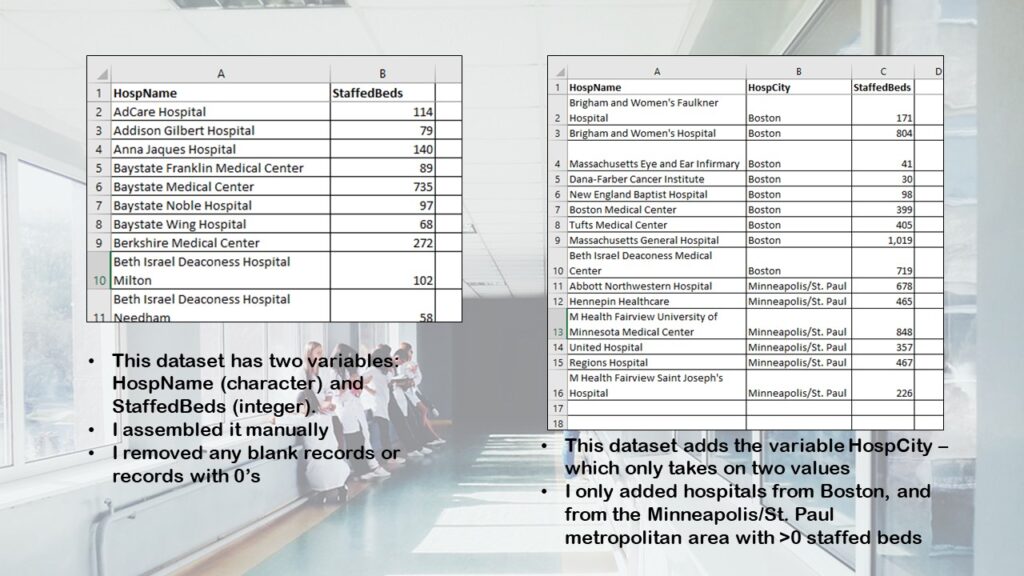
As you can see, there are two small datasets.
- The first dataset has all the Massachusetts hospitals that had nonzero StaffedBeds that I found when I went to AHD.com (they continuously update the data). Sometimes hospitals report 0 staffed beds due to delayed reporting or other reasons. I assembled this dataset to give you an idea of making a box plot of a population of values, so I cheated and removed the missing data, rather than trying to do anything scientific about it.
- The second dataset has only the hospitals with nonzero StaffedBeds from two metropolitan areas: Boston and Minneapolis/St. Paul. This was because I wanted to demonstrate a realistic comparison scenario. These two metro areas are about the same size population-wise (Boston is a little bigger). I had to look up the Minnesota hospitals for their data, and combine them into one HospCity level (so you won’t see this value in the original data from AHD.com).
Making Box Plots in Base R: Quick ‘n’ Dirty
As you will see in the code on Github, I named the first dataset MAHosp, and after reading it into R GUI, all I had to do to get a base R box plot was this command:
boxplot(MAHosp$StaffedBeds)
…which produced this:
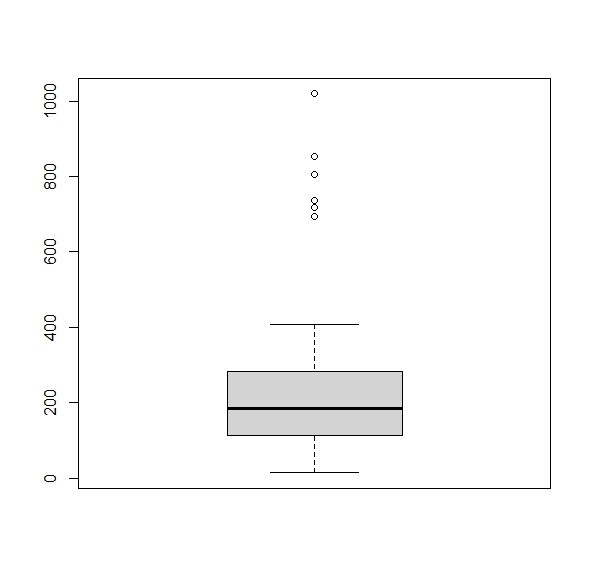
Notice how it is pretty sparse, and also, it is in a vertical orientation. This is what I normally do when I am speeding through data analytics and I just want to quickly know the distribution of a variable.
But base R also offers the opportunity to “deck out” your box plot. Here is just one example if what you can do to make it fancier and prettier:
boxplot(MAHosp$StaffedBeds, col = c("gold"), xlab = c("Massachusetts Hospitals"), ylab = c("Staffed Beds"), notch = TRUE)
…which produced this:
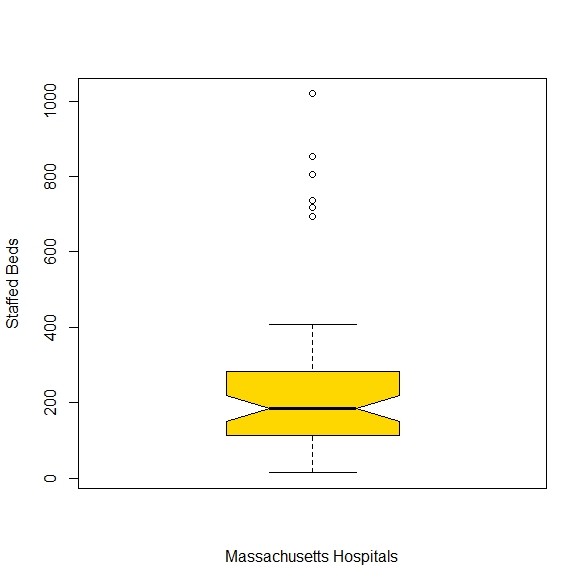
Maybe I went a little crazy with that notch = TRUE thing, but I wanted to show you the capabilities of base R. When I first started with R, I observed that many R users would “put down” plotting in base R, and say it was stupid to do. Why do that when you can use the ggplot2 package?
But I admit, I was very intimidated by the ggplot2 package. ggplot2 has its own set of syntax – think “data steps” in SAS. So it is very hard until you get used to the syntax, and how you have to do things in a certain order – again, not unlike data steps in SAS! But once you get used to ggplot2, you will realize it is extremely extensible, and the resulting plots are readily customizable. ggplot2 can do things that are way more amazing than base R – but this advanced visualization tool is only really necessary in certain circumstances.
Making Box Plots in gglot2
So let’s start by making the most basic box plot possible in ggplot2 with our MAHosp dataset and StaffedBeds variable. That would be this code here:
ggplot(data = MAHosp, aes(x = StaffedBeds)) + geom_boxplot()
…which produces this:
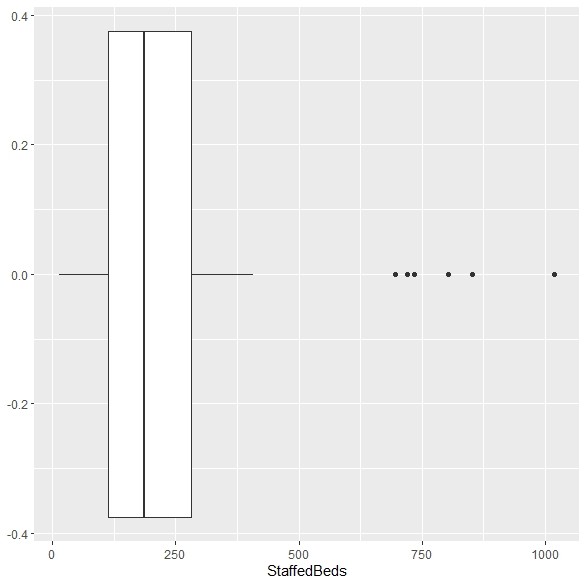
In a way, comparing base R’s vanilla box plot to ggplot2’s is instructive. I consider ggplot2 a snob. The base R plot is nice and neat and easy to interpret, even if it is sparse. But the vanilla ggplot2 plot is so over-the-top. So extravagant, high maintenance, and spoiled, expecting to be accessorized with expensive labels and formats. It’s like the plot washed its face and sat down on the toilet, expecting you to apply make-up. It really could not even go out to Publix or a predatory journal looking like that!
I’m more of a base R person myself. No matter what, I always look presentable, even without axis labels and a title (or make-up, for that matter). But then, kind of like base R, I always look pretty much the same. I make a better fashion designer than a model. And ggplot2 is for models – it’s for really showcasing your results, and putting them on display.
One point of contention I have is that the ggplot2 box plot comes out horizontally, and in healthcare, we usually put them vertically (for whatever reason). So in my data science makeover of my ggplot2 vanilla box plot, I use coordflip() to get it standing up straight! Here is the code:
ggplot(data = MAHosp, aes(x = StaffedBeds)) + geom_boxplot(fill = "pink") + xlab(c("Staffed Beds")) + ylab(c("Massachusetts Hospitals")) + coord_flip() + theme_classic()
…and here is the plot:
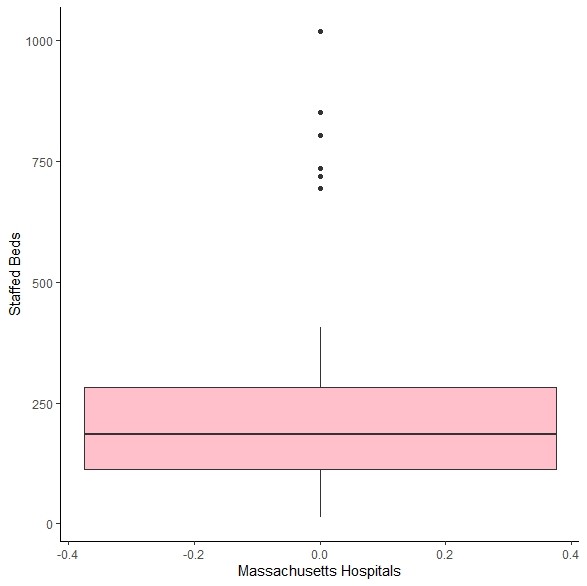
Observe the classic ggplot2 syntax, which was what confused me at first. I try to remember that aes apparently stands for “aesthetic”, and it tends to take the variable you are graphing as the main argument. But the trick is that if you don’t add a plus at the end of the line and then on the next line, declare some sort of shape (in our case, it’s geom_boxplot), you won’t see anything. You can validate this claim by just running the first line of ggplot2 code above without the plus. It will show you a blank graph.
Making Box Plots to Compare Groups
Most of the time, in healthcare, when we make box plots, we are trying to compare the distribution of some quantitative variable between various groups. For example, I will typically find myself making box plots of a certain lab value where I am comparing different diagnostic groups of patients.
The problem is that it’s not always obvious to know when making box plots how to modify the code above to get group comparisons. To get you a fair comparison, I assembled the CityCompare dataset as I described above, and imported that into R GUI (as you will see in the Github code).
boxplot(StaffedBeds ~ HospCity, data = CityCompare, col = c("pink", "gold"), xlab = c("Compare Cities"), ylab = c("Staffed Beds"))
You will see the main changes are adding the equation StaffedBeds ~ HospCity as the first argument, and adding another color so that R knows what color to make both the boxes. Here is the result:
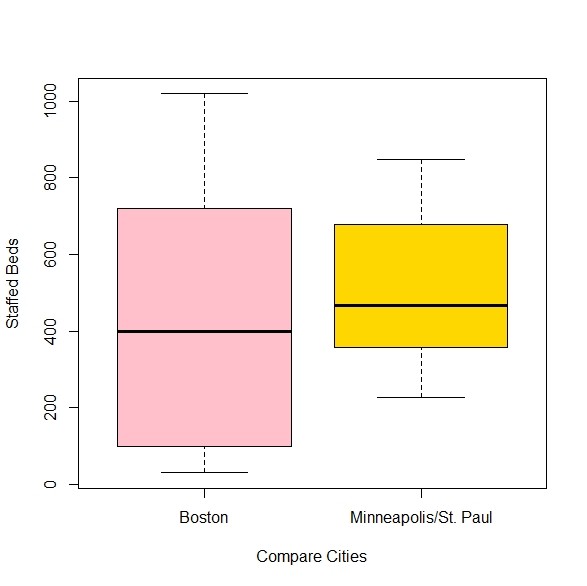
I am observing only now that ggplot2 is not big on the “whiskers” part of the box-and-whisker plot, but base R delivers. Let’s look at the ggplot2 code for the fancy comparison box plot.
ggplot(data = CityCompare, aes(x = StaffedBeds, y = HospCity)) + geom_boxplot(fill = c("pink", "gold")) + xlab(c("Staffed Beds")) + ylab(c("Compare Cities")) + coord_flip() + theme_classic()
Like with base R, we had to add another color, but in this case, we added the color to the geom_boxplot argument. Here is the plot:
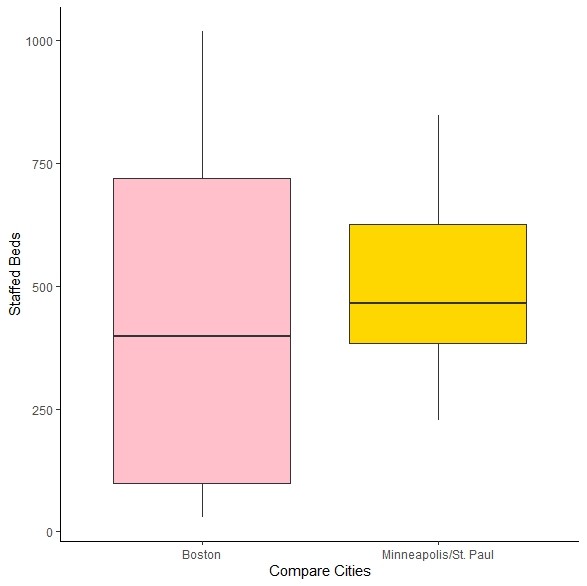
So in summary:
Is ggplot2 super complicated? Yes. But is it easy to Google for code and just kludge together something totally sexy in ggplot2? Dangerously, the answer to that question is also, “Yes”.
Making Box Plots in R: How I Do it
This is how I do it:
- If I am busy programming and I need to know the distribution of a variable, it’s base R all the way. I just throw up a box plot, or a set of comparison box plots.
- But if the project gets involved, and I think I’m going to have to actually present these box plots in a report, I switch and rebuild them in ggplot2. That way, I can keep using the ggplot2 tools – including ggsave – and all the packages that connect to ggplot2 and work with its objects, like the likert package.
Updated March 31, 2022. Added video April 2, 2022. Added banners March 6, 2023.
Read all of our data science blog posts!
Making box plots in R affords you many different approaches and features. My blog post will show you easy ways to use both base R and ggplot2 to make box plots as you are proceeding with your data science projects.