Joins in base R are more natural to you if you are not regularly programming in SQL. If you are used to SQL, then you will likely prefer not to do your dataframe joins in base R. Instead, you will more likely groove with the R package dplyr that allows you to make joins between dataframes using more SQL-like code.
But for a lot of programmers like me in public health (and other domains that use SAS), base R is more natural than dplyr. Therefore, we are more likely to gravitate towards doing our joins in base R. Also, dplyr uses “tibbles”, not regular dataframes, and I prefer dataframes, so I usually stick with base R for data management.
But I like joins! In SAS, we do joins using the old “sort, sort, merge” routine. If we want to do left or right joins, or inner or outer joins, we have to adjust our merge code. In base R, joins can be approached in a similar way as in SAS – but luckily, you don’t have to do the “sort, sort” first!
Joins in Base R: NHANES Use Case
Right here, we are going to start with some code that is a repeat from this blog post about joining NHANES data. You can download the code from GitHub if you are interested. The blog post explains the back story on the NHANES data, and directs you online where you can download the datasets used in this example.
Setting Up the Data
The NHANES datasets are in SAS XPT format, but If you use the foreign package in R, you can easily unpack them into the R environment, which we will do. As described in the blog post, the NHANES data are federated. Therefore, they have to be joined together to make an analytic dataset. The way the business rules go, the demographic dataset (P_DEMO.XPT) is essentially the “denominator file”. It has one record for every respondent in the data. The primary key is SEQN, and SEQN is a foreign key in all the other datasets. Therefore, to build out a dataset for analysis, it is necessary to do a series of left joins onto demographic dataset.
As you can see below, I import the demographic data into a dataset named demo_a. I also import a dataset with oral health examination data called P_OHXDEN.XPT into R, and I name the dataset called dent_a.
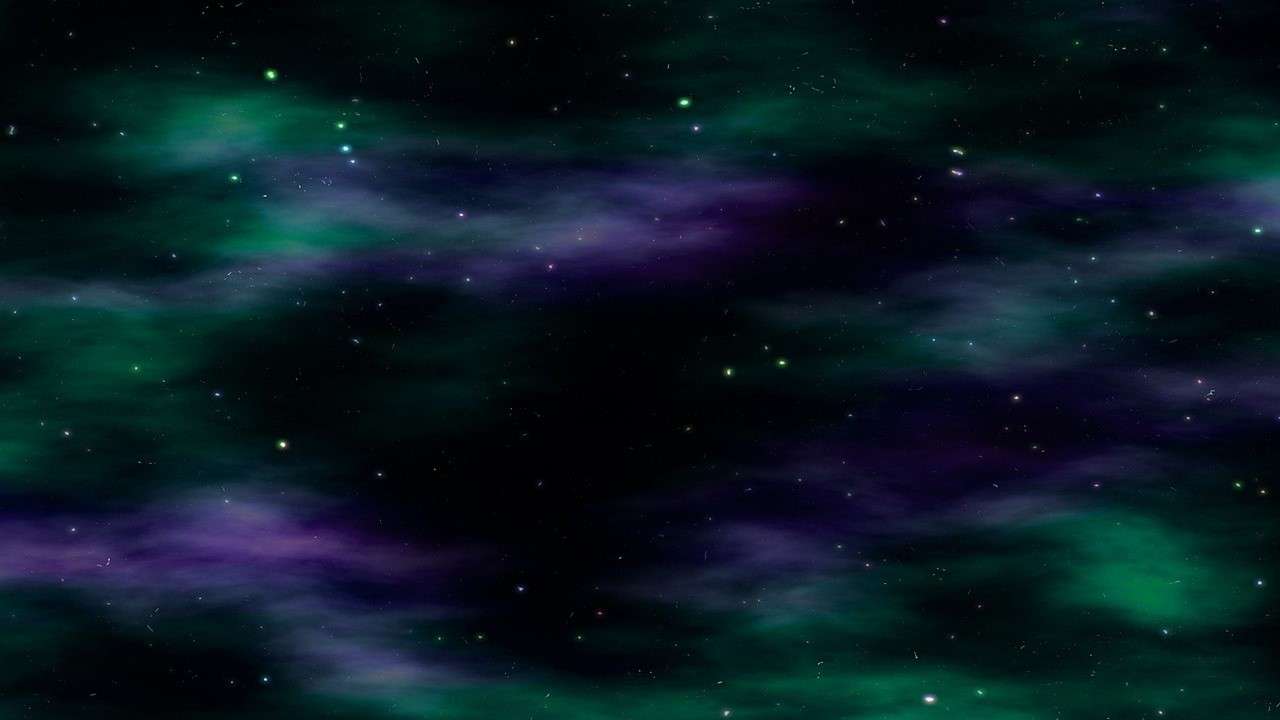
library(foreign) demo_a <- read.xport("P_DEMO.XPT") dent_a <- read.xport("P_OHXDEN.XPT")
The preceding code imports all the variables in each of the source datasets, but we don’t want all the variables. In fact, as described in the blog post, we know we just one two variables from each dataset: SEQN (the primary key), DMDYRUSZ (variable indicating length of time living in the United States) from the demographics, and OHXIMP (variable indicating if respondent has a dental implant) from the oral health dataset. Here is the code I used:
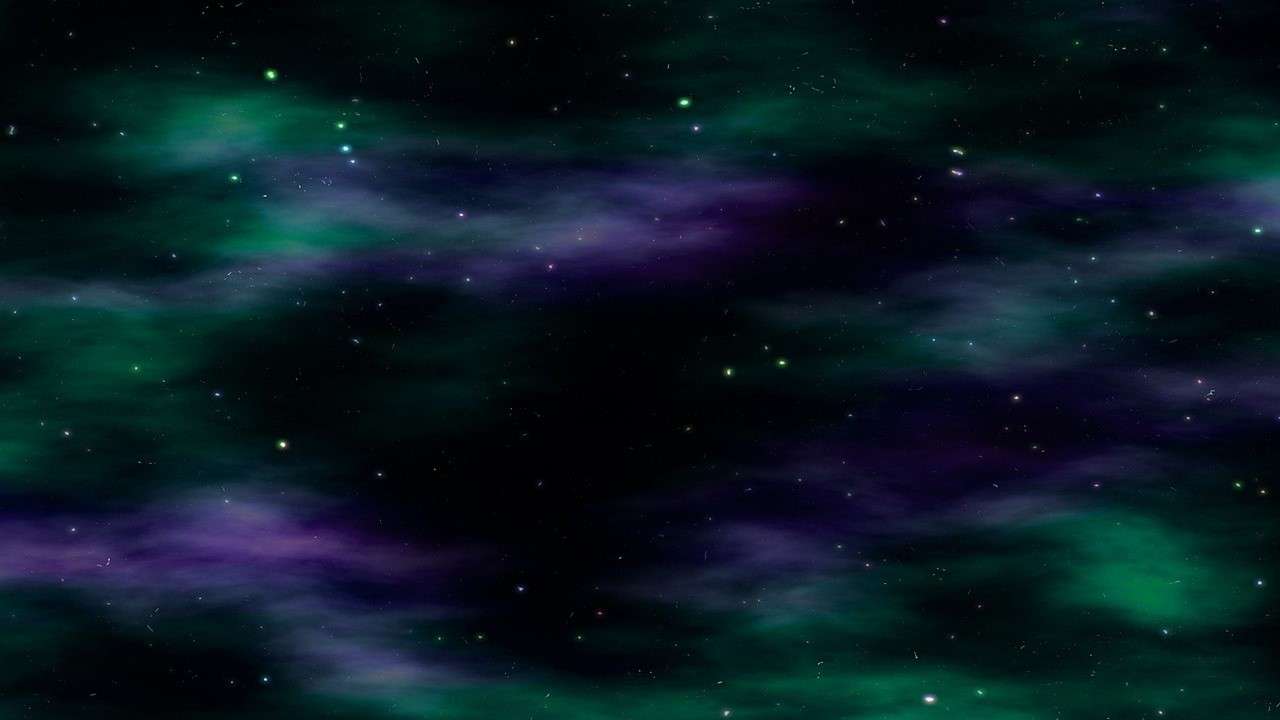
keep_demo <- c("SEQN", "DMDYRUSZ") keep_dent <- c("SEQN", "OHXIMP") nrow(demo_a) demo_b <- demo_a[keep_demo] nrow(demo_b) ncol(demo_b) colnames(demo_b) nrow(dent_a) dent_b <- dent_a[keep_dent] nrow(dent_b) ncol(dent_b) colnames(dent_b)
As you can see, to trim off the columns I do not want, I create a vector containing the list of variable names for variables I want to keep. I name the vectors after the dataset to which they are referring (e.g., keep_dent). Then, I use the vector name in brackets to trim off the columns I don’t want and rename the dataset. Using the keep_dent vector against the original dataset I imported and named dent_a, I create dent_b. I also created demo_b the same way – by just keeping the variables I wanted from the demographic dataset.
Joins in Base R: Correct Left Join
Now we will start by correctly joining dent_b onto demo_b using base R to execute a left join. If you left join dent_b onto demo_b, you are telling R that you want it to keep records with all the SEQNs from demo_b, and include data from records into the merged dataset that match up from dent_b. If there are no records that match up with dent_b from a SEQN in demo_b, then those values in the resulting merged dataset will be NA (empty). But, at least the data from demo_b will be in the merged data, and you will be able to tell which records had no matches in dent_b because those variables will be blank.
The reason why we call it a “left join” is we say the datasets in this order when doing the merge command: demo_b, then dent_b. See how demo_b is on the left of dent_b when we say it in that order? The merge command in R assumes that by putting demo_b first, we are designating it as the “x” dataframe. The “y” dataframe is dent_b, because it comes next.
So, in the next code, we first check the number of rows in demo_b with nrow. Given the governance policies of how the NHANES datasets are related, the business rule is our merged dataframe should have the same number of records as demo_b. If it doesn’t, we did something wrong, so we should check that number first – before the merge.
Next, we create the new merged dataframe as merged_a. In the merge command, to execute a left join onto demo_b, we state demo_b first and dent_b second. We specify the primary key, which is SEQN. Then, to make sure we get a left join, we add the option all.x = TRUE. This tells R to keep all the records in the x dataframe (demo_b), and only matching ones in the other ones.
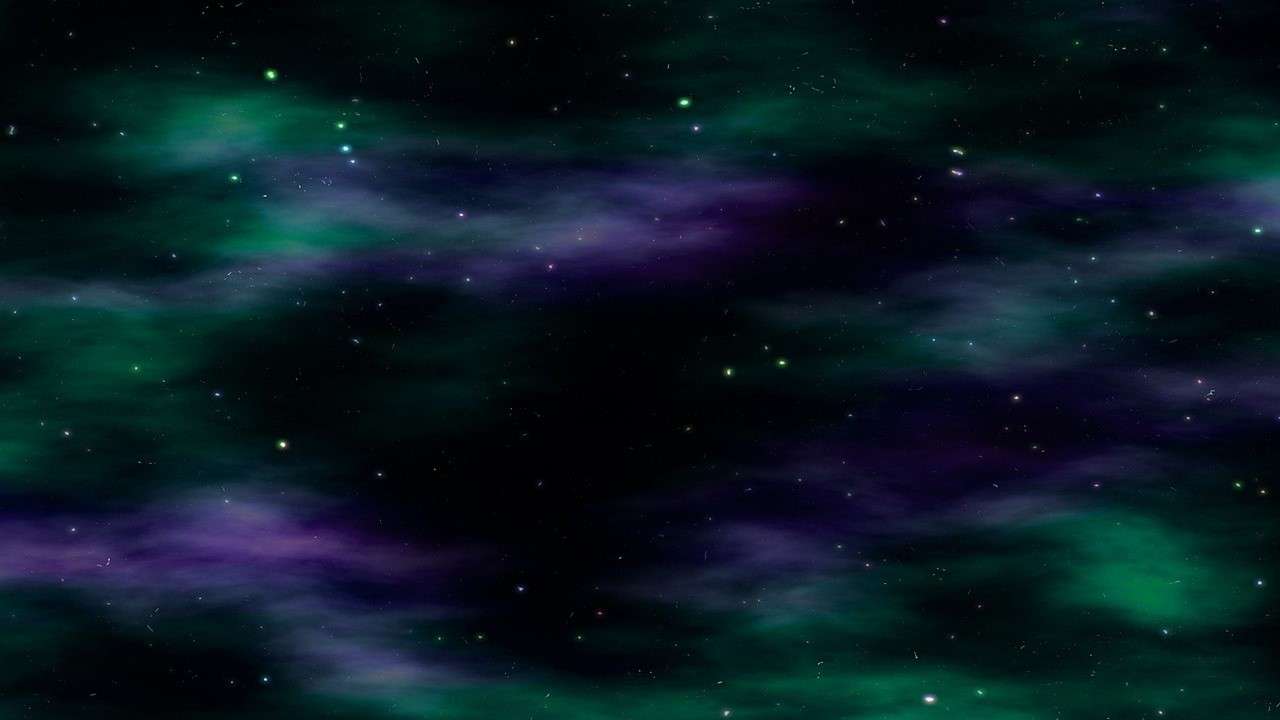
nrow(demo_b) merged_a <- merge(demo_b, dent_b, by = c("SEQN"), all.x=TRUE) nrow(merged_a)
So we use merge and all.x to left join dent_b onto demo_b and create merged_a. You will notice I checked the number of rows to ensure the left join worked, and it looks like it did.
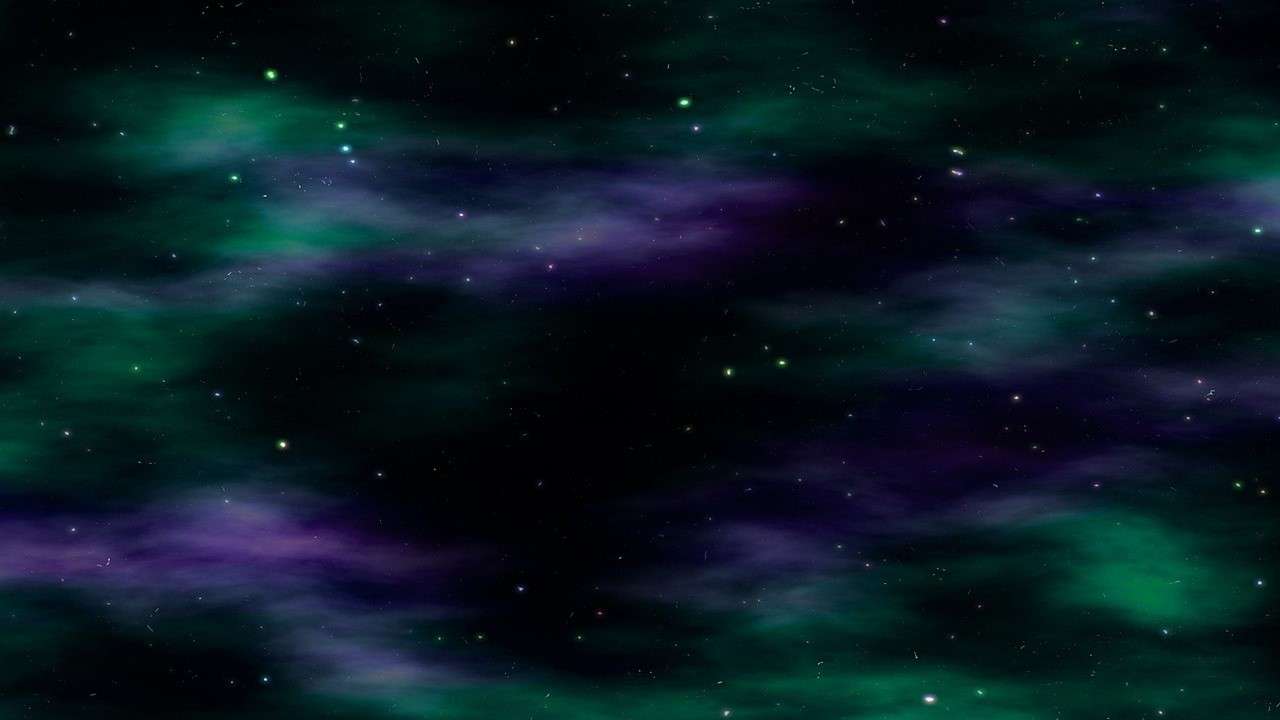
> nrow(demo_b) [1] 15560 > merged_a <- merge(demo_b, dent_b, by = c("SEQN"), all.x=TRUE)
> nrow(merged_a) [1] 15560
Notice that the demographic table says that the total universe of possible respondents is 15,560 in this dataset. But even though all of those records joined (because we forced them), we don’t know if the variables we wanted from dent_b are available for all the records. In fact, we know many will be missing. So we have to do a frequency table looking at the variable OHXIMP from dent_b variables and values of NA.
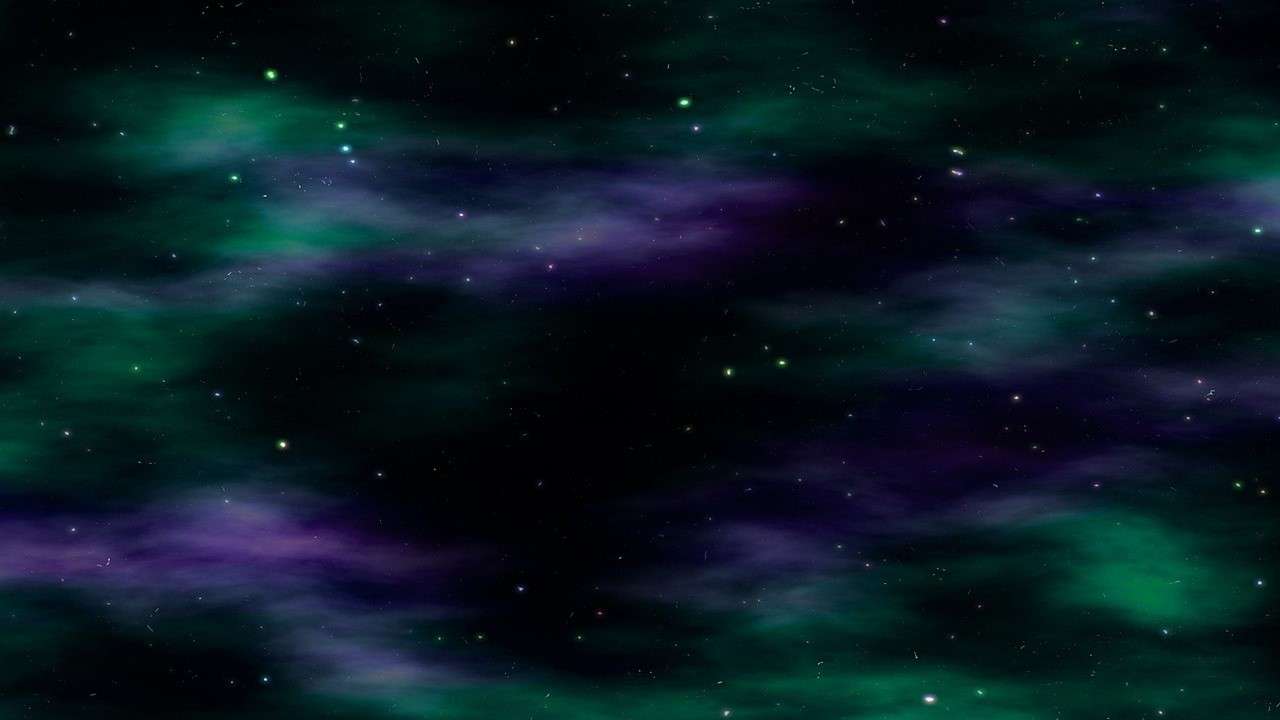
Code: table(merged_a$OHXIMP, useNA = c("always")) Output: 1 2 NA 358 9390 5812
As you can see, the records coded with 1 and 2 represent data joined on from OHXIMP. The 5,812 that didn’t join represent missing data in OHXIMP.
The issue with NHANES (and a lot of other datasets) is that the demographic dataset is the only one that has a full list of respondents. Only a subset of those respondents are also in the oral health dataset. A different subset would be in the smoking questionnaire dataset. So if we don’t always join all these datasets with demographics on the left – with a properly-executed left join – we will lose records.
Now, I will go over a few common mistakes.
Mistake #1: Accidentally Doing a Right Join
Let’s do this join again, but this time, we will change part of the code. We will keep the datasets in the merge command in the same order (demo_b first, followed by dent_b), but this time, instead of setting all.x = TRUE, we will set all.y = TRUE.
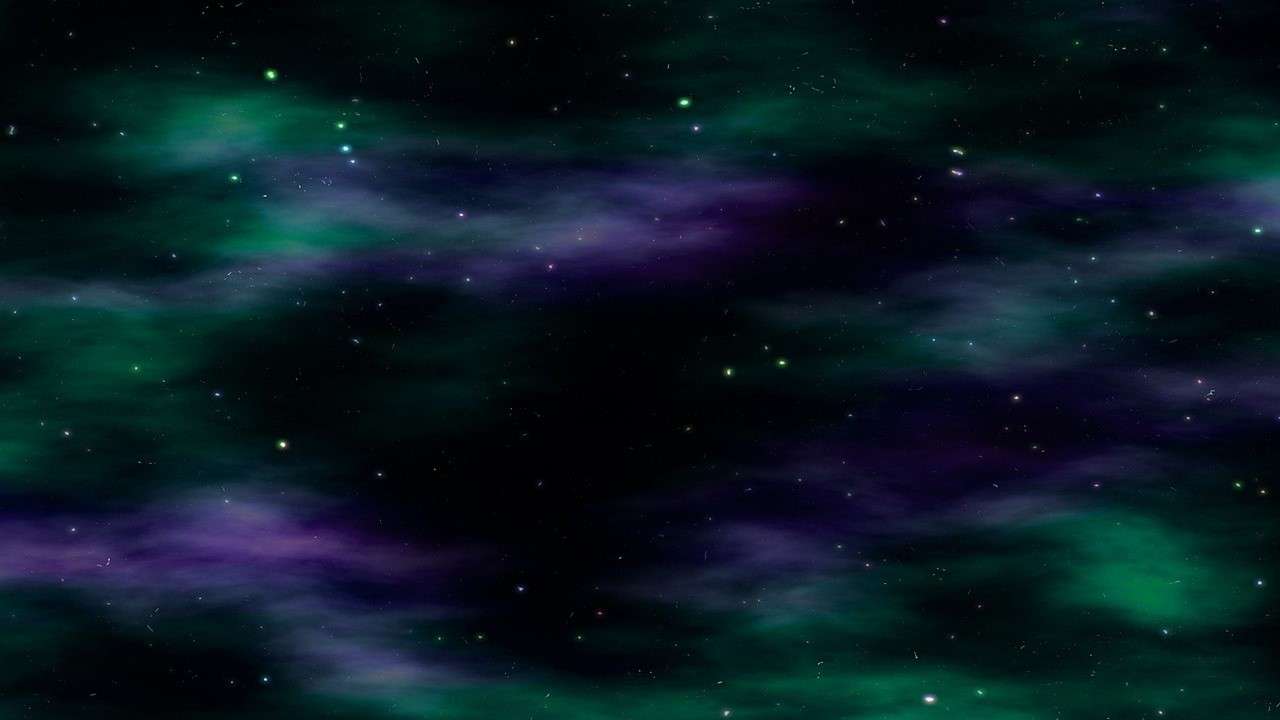
nrow(demo_b) merged_a <- merge(demo_b, dent_b, by = c("SEQN"), all.y=TRUE) nrow(merged_a)
By doing this, we are telling R to keep all the SEQNs in dent_b, and only keep the ones from demo_b that matched dent_b. This limits the universe of the retrieved records to only the respondents who underwent the oral health exam, which are in dent_b.
This mistake is apparent when looking at the output on the console:
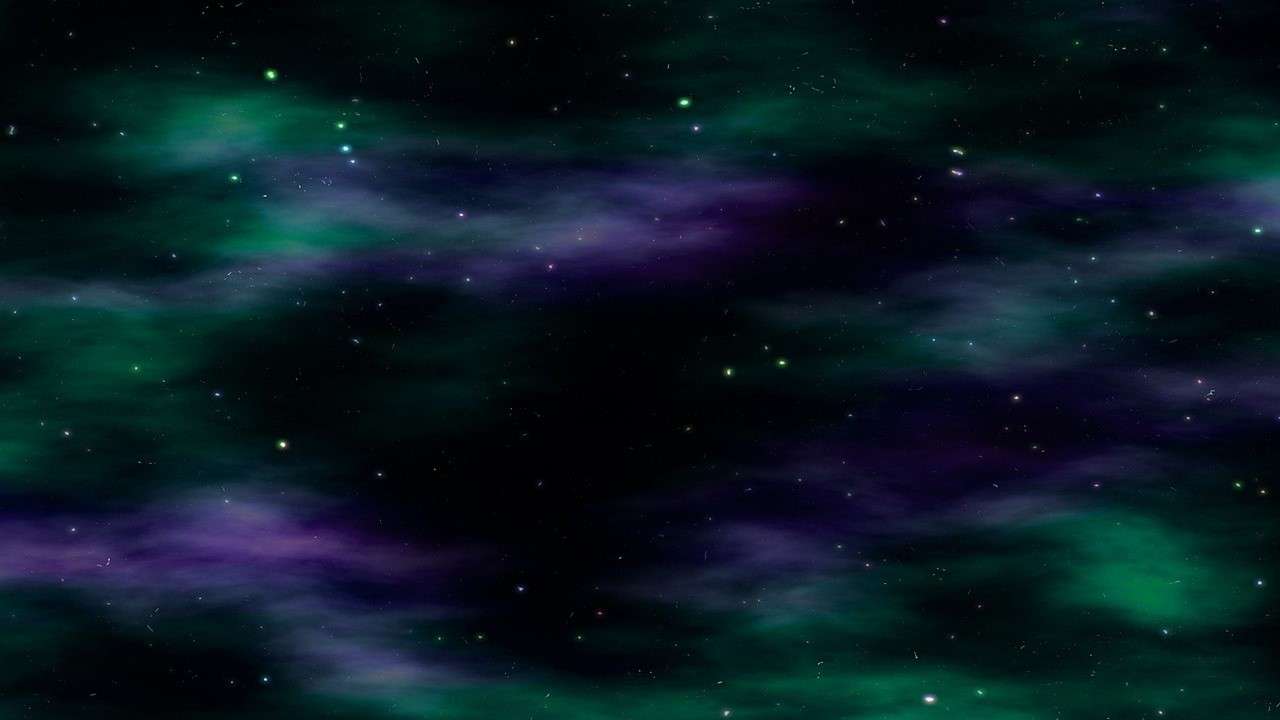
> nrow(demo_b) [1] 15560 > merged_a <- merge(demo_b, dent_b, by = c("SEQN"), all.y=TRUE)
> nrow(merged_a) [1] 13772
Clearly, the dataset has been reduced from 15,560 records to 13,772 – not good. We are losing records – all because we said all.y instead of all.x.
Mistake #2: Putting the Wrong Dataframe First
You can make exactly the same mistake as the one we just did by keeping all.x = TRUE the way it is, and just putting the dataframes in the merge command in the wrong order, with dent_b first and demo_b second. Here is the code with the number of rows from the console.
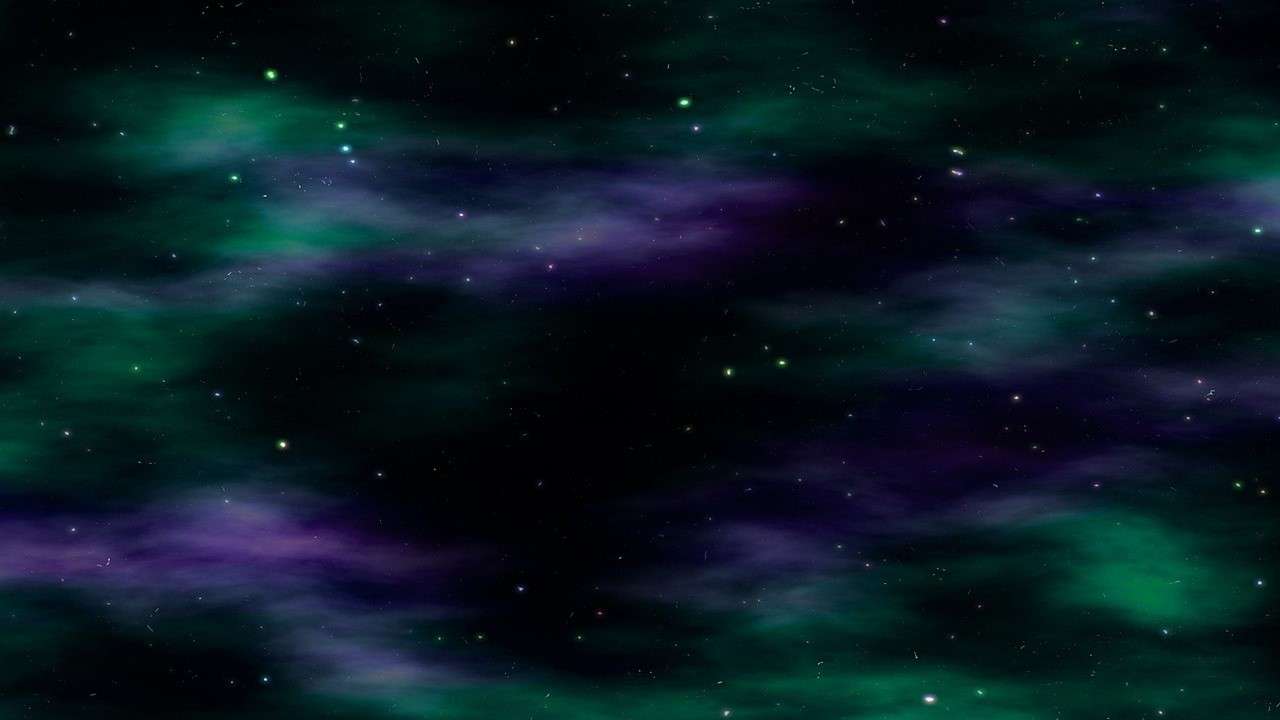
> nrow(demo_b) [1] 15560 > merged_a <- merge(dent_b, demo_b, by = c("SEQN"), all.x=TRUE)
> nrow(merged_a) [1] 13772
As a convention, because the demographics represents the entire universe of SEQNs, it should always be stated first, and serve as the left side of a left join through each merge step. As more and more dataframes are patched on, we should not see the number of rows ever change from that 15,560 number. That’s because NHANES is a cross-sectional dataset, so their should be only a one-to-one relationship between the demographic dataset and the others.
Mistake #3: Accidentally Doing an Inner Join
When you do an inner join, you are telling R to only keep the records in the resulting dataset that are in both datasets (demo_b and dent_b). In our case, that would essentially give us the same results as right joining dent_b onto demo_b. However, that is not always the case – it depends on the business rules of the underlying data.
This is why we need to add the all.x = TRUE option – to prevent R from doing an inner join, and not a left join. Here is what happens if we leave that option out.
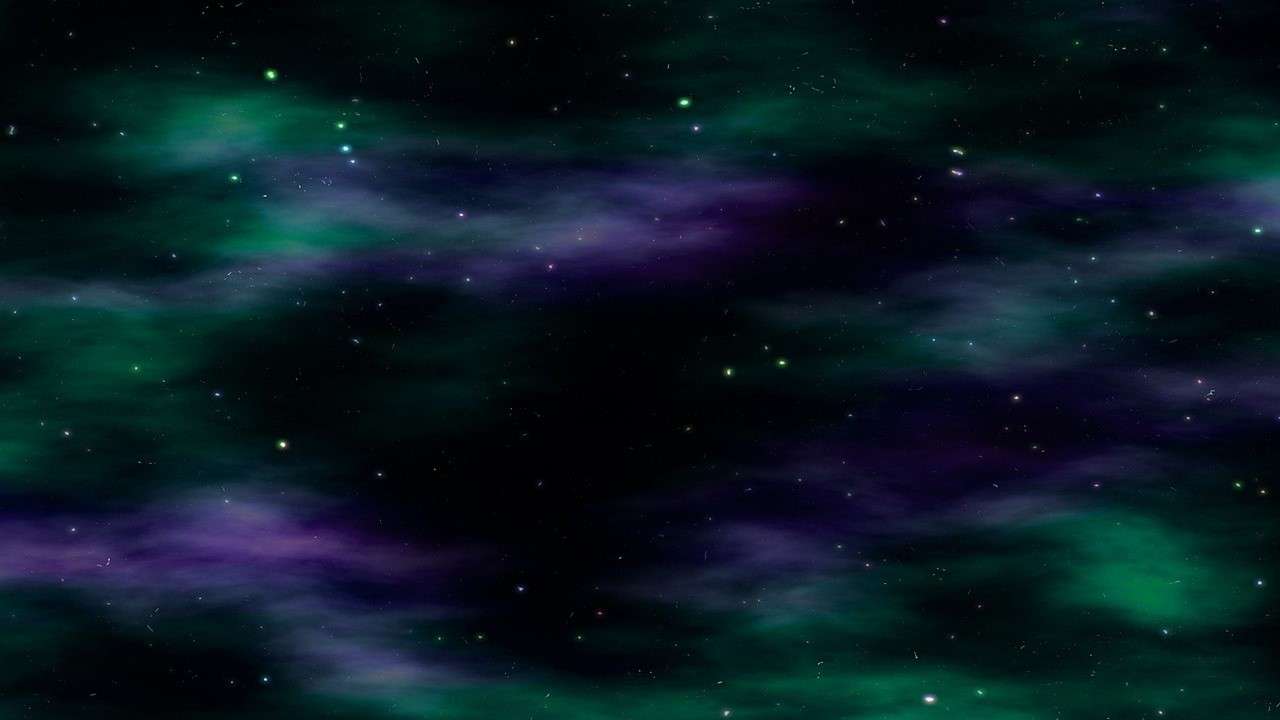
> nrow(demo_b) [1] 15560 > merged_a <- merge(demo_b, dent_b, by = c("SEQN"))
> nrow(merged_a) [1] 13772
Again, we see that we lose records, as merged_a only has 13,772 records, not the 15,560 we expect.
Joins in Base R: Be Careful!
Just like when merging datasets in any statistical language, you have to be careful if you want to use base R to join datasets. First, make sure you understand the underlying business rules before you start joining the datasets. Next, make sure you regularly look at number of rows in datasets before and after merges to make sure you are not losing data (or having any other join issues). And, if you don’t know R and just want to learn it, try my LinkedIn Learning course in “Descriptive Healthcare Analytics in R”.
Added video December 4, 2023.
Read all of our data science blog posts!
Joins in base R must be executed properly or you will lose data. Read my tutorial on how to correctly execute left joins in base R.