It’s not uncommon that I encounter an organization that has never heard the term “data stewardship”, but rarely have I seen such an abject failure in data stewardship as was outlined in John Carryrou’s book, “Bad Blood”, which describes the rise and fall of startup Theranos. Theranos was supposedly making a new way to test blood in the lab, but the book shows that the scientists were in the dark about a lot of aspects of the product and the research going on at the organization, which resulted in – among other things – an abject failure in data stewardship.
How was Theranos an Abject Failure at Data Stewardship?
I mentioned in the first post in this series that junior laboratorians played a central role in taking down the Theranos hoax. One of them was Erika Cheung, who spoke about Theranos’s torture of data in her TED talk. I visualized one of the scenarios she described on a slide to better explain what happened.
To do quality control in a lab, you are often given a sample and told what concentration it is, and then you put it through your equipment and test it, and see if it gets the right answer. Cheung describes in her TED talk how she was asked to test a sample of that had a concentration of 0.2 TPSA on the new Theranos equipment (TPSA is a lab run for prostate cancer-related inquiries). Since we knew the concentration already, every lab run should have had a result that was close to 0.2. Instead, she reported some pretty wacky results, which I visualized in this chart.
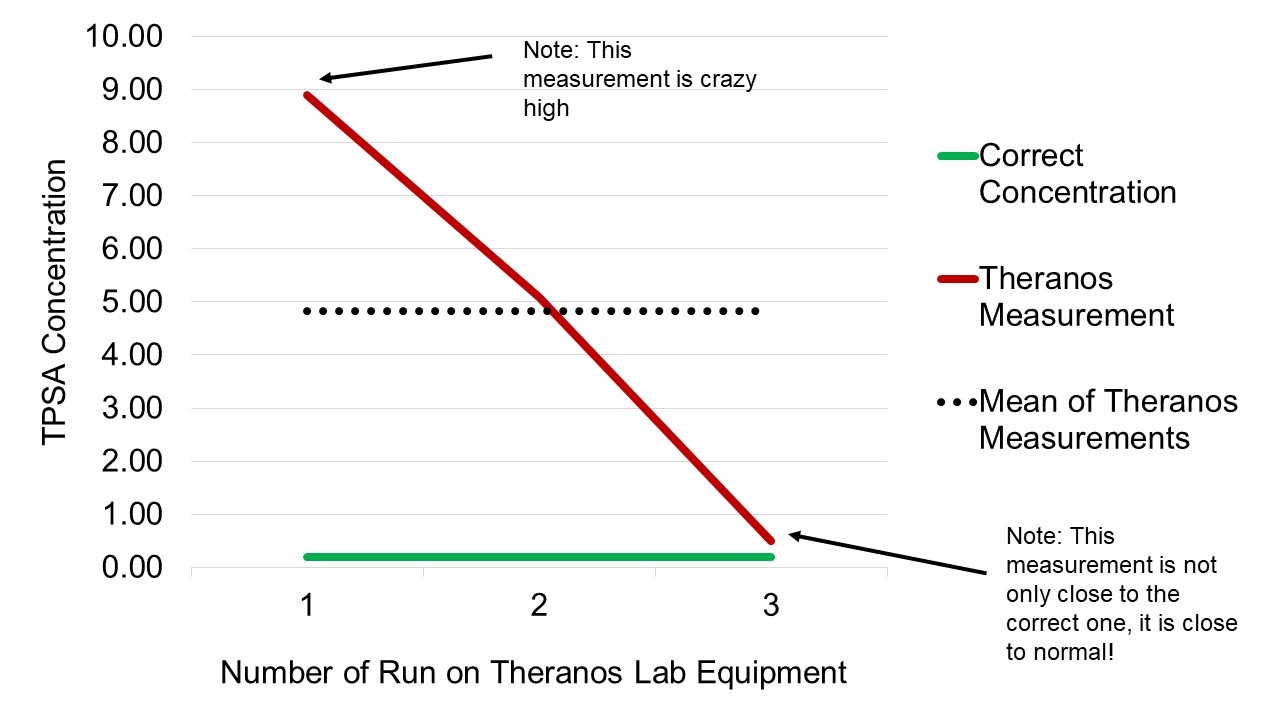
As you can see by the chart, Theranos’s equipment was producing results that were all over the place. Theoretically, all of these widely discrepant results should have been carefully documented in data.
But now we get to where Theranos was a real abject failure at data stewardship. Cheung went on to tell how when regulators provided a sample of a known concentration to Theranos to test and then provide the results back to them as part of quality control, Theranos essentially cheated. What they did is split the sample, and tested part of it on the Theranos equipment, and part of it on commercial lab equipment already approved and in use that they purchased. Cheung said that predictably, the commercial equipment produced the same result in each run, and the Theranos equipment was all over the place. So what did Theranos do?
They lied. They submitted the data from the commercial lab equipment and said it was from their equipment.
Preventing Such an Abject Failure of Data Stewardship
Theranos is an interesting case, because they were actually lying intentionally, and took steps to try to hide this from the scientists. Of course, people in Cheung’s position learned of the lies – but Carryrou describes how Theranos’s CEO Elizabeth Holmes worked ruthlessly to compartmentalize scientists from each other so they could not share notes.
Hopefully, everyone reading this already realizes that keeping scientific teams who are supposed to be developing the same product from speaking to each other will result in some pretty bad science. Because not everyone knew about the lies, only certain people knew that it was a hoax. Among those, only a few people spoke out – like Cheung. That’s eventually how the hoax was discovered. But something like this that ended up harming patients should have been prevented.
As I mentioned earlier, an important way to prevent an abject failure of data stewardship is to make sure that communication lines are as open as possible among the scientists on different teams. I’m a strong proponent of creating data curation files that all scientists can understand. I’ll give some examples here.
The data that come out of lab equipment are often formatted in a really terrible way for data scientists (e.g., crosstabs format, unstructured data, etc.). Therefore, I have to document how I transform the data for analysis – and make sure I understand all the data points associated with each run. I need to keep all the samples in the run straight – because, as you can tell, some of them have known concentrations and I have to keep track of that.
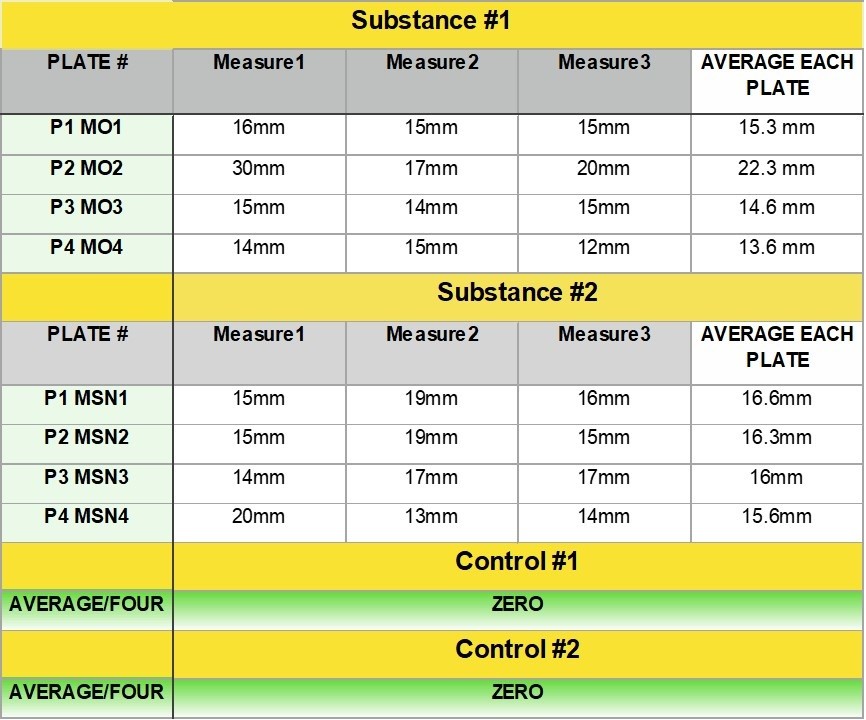
But the market researchers and epidemiologists (like me) have surveys that might be part of the same study as the lab data. I remember working on a study of caffeine metabolism in older individuals, and we had lab data as well as survey data. All of this needs to be documented so that all the different type of researchers stay on the same page. That’s why big, long epidemiologic studies such as the Atherosclerosis Risk in Communities (ARIC) study have so much data documentation. Careful data documentation and data curation are excellent approaches to improving research management, and preventing an abject failure of data stewardship.
Blog menu added July 19, 2021. Banners updated June 16, 2023.
Read all posts in the series!
The book “Bad Blood” describes the fall of startup unicorn Theranos, but also provides insight into the company’s abject failure at data stewardship, which I talk about in this blog post.