I took this LinkedIn Learning course in explainable AI after learning about XAI at a SAS webinar I attended. Perhaps the most important takeaway from this course is that most of the AI we are subjected to right now is NOT explainable. Explain to me why I search for something online for one of my customers – let’s say some therapeutic product for babies – and then later, I get e-mails, pop-ups, and online ads for that. Anyone who knows me knows that there are no babies anywhere near me, so that is definitely wasted marketing.
These crazy companies need to take this course in explainable AI.
Why would they think that I, an old data crone, would actually be purchasing baby products? Well, these “crazy companies” now want to know that answer, too, because they are beginning to wonder if they really want to keep overspending on selling baby products to someone (hopefully) going into menopause. Also, they worry about the legal risks of AI algorithms recommending different things for inexplicable reasons. Basically, people are sick of AI being a black box – so enter XAI.
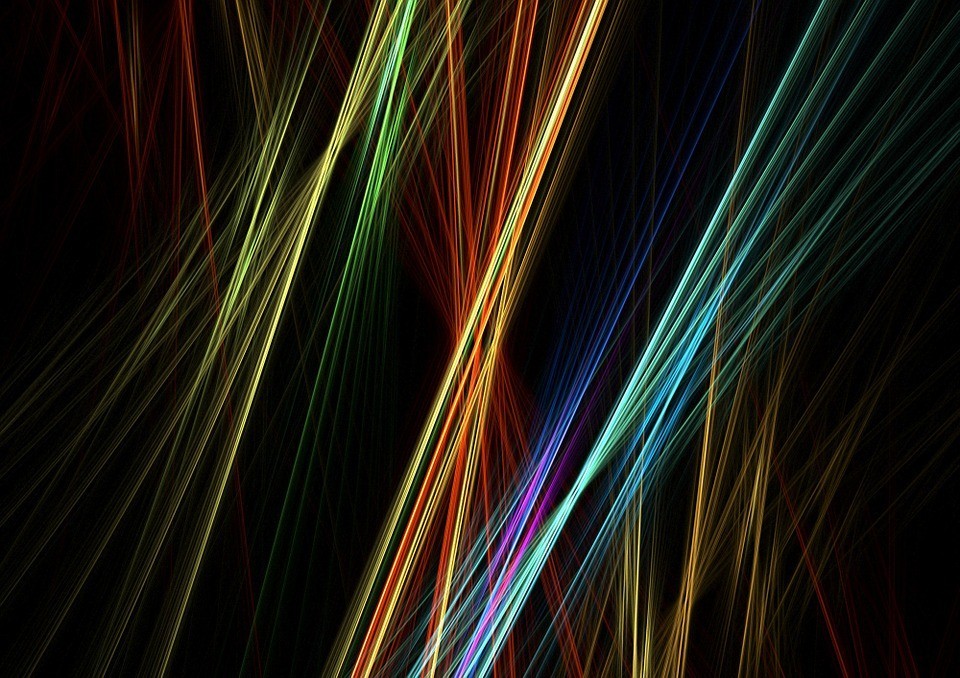
Definitions in this Course in Explainable AI (XAI)
The instructor, Aki Ohashi, directs Business Development at the Palo Alto Research Center (PARC), a historical technology research center that brought us many innovations over the years. So I trust what he says in this course in explainable AI, but I was admittedly shocked to learn from him that XAI is currently in its infancy. The reason why I was shocked is that I do a lot of logistic regression in public health, and we absolutely have to be able to explain our models. If you can’t explain the model to yourself, how do you know if it’s running properly?
Ohashi described three approaches used for XAI right now:
LIME (Local Interpretable Model-Agnostic Explanations)
- Does post-hoc analysis to figure out why the model said what it did. So, keep in mind Type I error.
- Is agnostic of the type of model used to make the prediction. That way, you can use it on any type of AI model.
- You perturb the inputs and see what happens to the outputs. That way you can play with the model. I like to do this on an Excel sheet with my logistic regression equations.
RETAIN (Reverse Time Attention): Developed at Georgia Tech for predicting heart attacks, so already, I pretty much reject it. The entire cardiovascular epidemiology field excluded women as participants and researchers for so long, I do not believe anything developed based on that line of inquiry, because it’s missing a good chunk of our population. Besides, the data in healthcare are messy, and should not really be used for purposes like these.
LRP (layer-wise relevance propagation): Works a little like LIME in that it does a post-hoc analysis about what inputs had the most influence, but unlike LIME, uses neural networks/deep learning approaches.
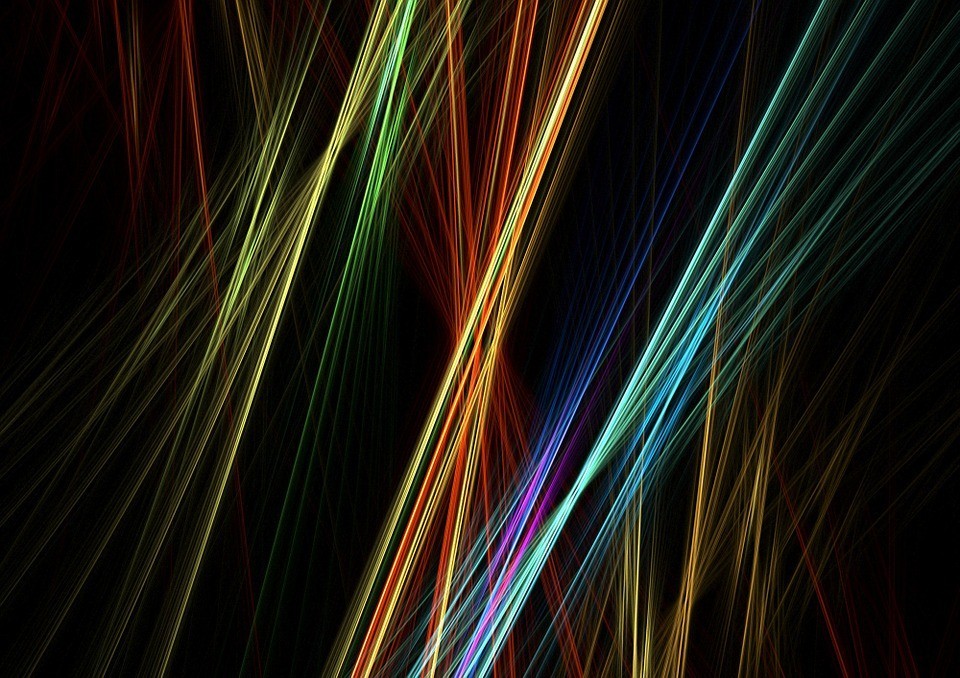
Published December 14, 2020. Big data graphic by DARPA, available here.
We experience artificial intelligence all the time on the internet in terms of friend suggestions on social media, internet ads that reflect what we have been searching for, and “smart” recommendations from online stores. But the reality is that even the people who build those formulas cannot usually explain why you were shown a certain suggestion or ad. That’s what “explainable AI”, or XAI, is all about!